
无论您是有气术家的家,嘈杂的社区,还是降低能源消耗和成本的愿望,来自Indow的室内窗口插件都是一个明确的选择。
无论您的窗口问题是什么,Adow Windows Compression-Fit插入物manbetx客户端应用下载与您现有的窗框一起使用,因此无需花费大量现金来代替您的窗户。使用专有软件制成,我们的插入物的尺寸非常准确,可以在不使用安装硬件的情况下安装,并且在安装一旦安装后,几乎消失了。
随时与我们联系以获取免费估算,以在您的位置安装窗口插入!
创建舒适,安静的空间从未如此简单。Indow的Storm Window插入了冬季的寒冷草稿和夏季的热空气,以节省您全年的能源。另外,它们会减少外部噪音,并帮助您控制光线。
不要更换窗户。manbetx客户端应用下载选择定制设计的插入物以使其正确。
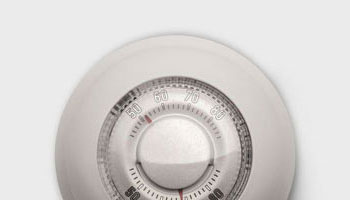
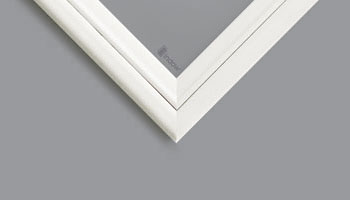
我们的风暴窗插入不是窗户。manbetx客户端应用下载它们不是外部风暴或磁性内部风暴窗。manbetx客户端应用下载取而代之的是,它们是定制设计的窗口插入物,只需按下现有窗框的内部,而无需硬件或安装括号。这意味着它们使您的原始窗户像新的窗户一样表现出色,从而改变了您的需求,manbetx客户端应用下载而无需替换窗户的巨额费用。
耐用的。在美国量身定制的易于安装。在线查看我们所有的风暴窗。manbetx客户端应用下载我们的丙烯酸窗口插入物中有一个柔软的硅胶压缩管,可产生紧密的密封。激光测量可确保它们精确地适合并牢固地安装在位。享受新窗户的所有好处,但采用更简单,定制的解决方案!manbetx客户端应用下载
查看所有大多大型产品manbetx手机客户端登入要了解有关我们的窗口插入如何帮助您克服窗户挑战的更多信息
一旦到达您的窗户,它们就可以立即舒适。manbetx客户端应用下载现在,您可以通过3个简单的步骤获得即时估算。

在家中度量

在线输入

查看您的估计
我们仍然检查您的测量结果,并将其准确的结果降至1/32“,但是此估计是找到即时舒适的第一步。
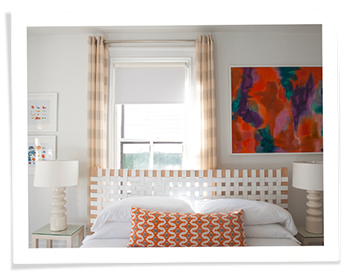